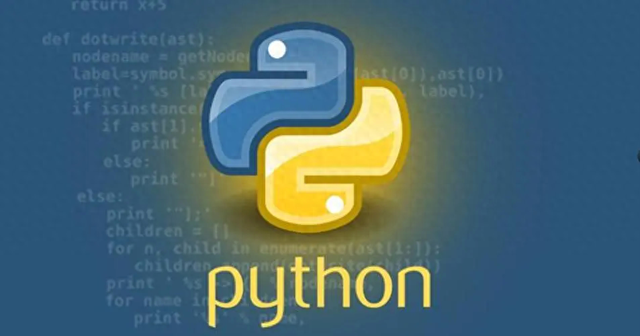
大家好,今天为大家分享一个强大的 Python 库 - neuralprophet。
Github地址:https://github.com/ourownstory/neural_prophet
时间序列数据在许多领域中都占据着重要地位,如金融、气象、销售等。对时间序列数据进行准确的预测可以帮助我们做出更明智的决策。而NeuralProphet库的出现为时间序列数据的预测带来了新的可能性。本文将深入探讨NeuralProphet库的使用方法、功能特性以及如何利用它进行时间序列数据的预测。
什么是NeuralProphet库?NeuralProphet是由Facebook开发的一个基于神经网络的时间序列预测库。它是Prophet库的进化版,结合了神经网络的强大能力和Prophet库的简单易用性。NeuralProphet库具有自适应性、灵活性和高性能的特点,可以用于预测各种类型的时间序列数据。
安装NeuralProphet库首先,需要安装NeuralProphet库。
可以通过pip安装NeuralProphet库:
pip install neuralprophet使用NeuralProphet库首先,需要加载时间序列数据。
import pandas as pd# 加载时间序列数据df = pd.read_csv('time_series_data.csv')NeuralProphet库使用了与Prophet库相似的数据格式,具有日期和目标列。
接下来,将数据拆分为训练集和测试集。
from neuralprophet import NeuralProphet# 拆分训练集和测试集train_df = df.iloc[:-100]test_df = df.iloc[-100:]然后,可以创建一个NeuralProphet模型并拟合训练集数据。
# 创建NeuralProphet模型model = NeuralProphet()# 拟合模型metrics = model.fit(train_df, freq='D')最后,可以使用训练好的模型来进行预测。
# 进行预测future = model.make_future_dataframe(train_df, periods=100)forecast = model.predict(future)NeuralProphet库的特性NeuralProphet库提供了许多功能特性,使其成为一个强大的时间序列预测工具。
1. 灵活的模型配置NeuralProphet库允许用户灵活地配置模型参数,以满足不同时间序列数据的预测需求。
model = NeuralProphet(n_changepoints=100, yearly_seasonality=True)2. 自动调整模型参数NeuralProphet库还提供了自动调整模型参数的功能,帮助用户优化模型性能。
model = NeuralProphet(auto_seasonalities=True)3. 多种评估指标NeuralProphet库支持多种评估指标,帮助用户评估模型的性能和准确度。
metrics = model.fit(train_df, validate_each_epoch=True)示例代码下面是一个完整的示例代码,演示了如何使用NeuralProphet库进行时间序列数据的预测。
import pandas as pdfrom neuralprophet import NeuralProphet# 加载时间序列数据df = pd.read_csv('time_series_data.csv')# 拆分训练集和测试集train_df = df.iloc[:-100]test_df = df.iloc[-100:]# 创建NeuralProphet模型model = NeuralProphet()# 拟合模型metrics = model.fit(train_df, freq='D')# 进行预测future = model.make_future_dataframe(train_df, periods=100)forecast = model.predict(future)高级特性和定制化NeuralProphet库还提供了许多高级特性和定制化选项,以满足不同场景下的需求。
季节性和假期效应NeuralProphet库支持对季节性和假期效应进行建模,以更准确地预测时间序列数据的变化。
model = NeuralProphet(seasonality_mode='multiplicative', holidays=holidays)多变量时间序列预测NeuralProphet库还支持多变量时间序列预测,可以同时考虑多个相关因素。
model = NeuralProphet(n_lags=10, num_hidden_layers=3)模型集成NeuralProphet库还支持模型集成的功能,可以通过组合多个模型来提高预测性能。
model_1 = NeuralProphet()model_2 = NeuralProphet()ensemble_model = EnsembleModel([model_1, model_2])高效的模型训练和预测NeuralProphet库的底层采用了高效的神经网络算法和优化技术,可以快速训练模型并进行预测。
metrics = model.fit(train_df, epochs=100, batch_size=64)可视化工具和模型解释NeuralProphet库提供了丰富的可视化工具和模型解释功能,帮助用户更好地理解模型的预测结果和内在机制。
model.plot(forecast)总结NeuralProphet库作为一个强大的时间序列预测工具,结合了神经网络的强大能力和Prophet库的简单易用性,为时间序列数据的预测带来了新的可能性。通过本文的介绍,了解了NeuralProphet库的基本用法、功能特性和高级选项。它不仅提供了灵活的模型配置、自动调整参数和多种评估指标,还支持多种高级特性和定制化选项,如季节性和假期效应建模、多变量时间序列预测以及模型集成。希望本文能够帮助大家更好地理解和应用NeuralProphet库,从而提高时间序列数据预测的准确度和效率。