Mistral AI社区发布了Mixtral 8x7B混合专家模型。这是一种具有开放权重的高质量稀疏专家混合模型 (SMoE)。 根据 Apache 2.0 许可。 Mixtral 在大多数基准测试中都优于 Llama 2 70B模型,推理速度提高了 6 倍。 特别是,它在大多数标准基准测试中优于 GPT3.5。
Mixtral 8x7B混合专家模型具有以下几个强大的功能:
上下文token数达到32k。可以处理英语、法语、意大利语、德语和西班牙语。代码生成方面具有强大的表现能力。可以微调为指令跟踪模型,在 MT-Bench 上获得 了8.3 分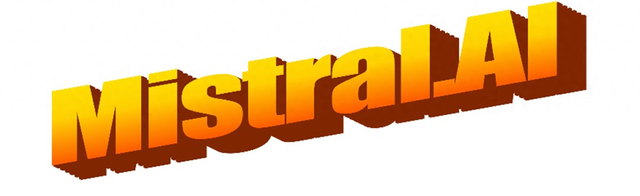
Mixtral 是一个稀疏的专家混合网络。 它是一个纯解码器模型,模型设计了8个专家组。 在每一层,对于每个输入token,神经网络模型选择其中的两个“专家”来处理输入token,并相加组合它们的输出。
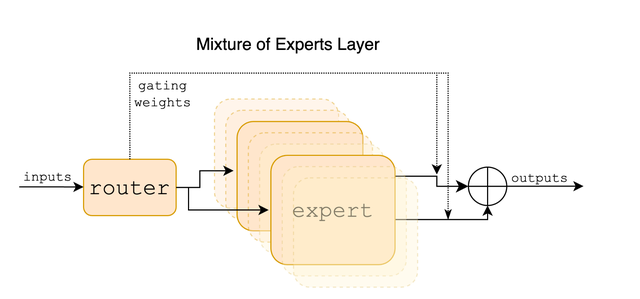
该技术增加了Mixtral模型的参数数量,同时控制了成本和延迟,因为Mixtral模型仅使用每个token总参数集的一小部分。 具体来说,Mixtral 共有 46.7B 个参数,但每个token仅使用 12.9B 个参数。 因此,它以与 12.9B 模型相同的速度和相同的成本处理输入并生成输出。
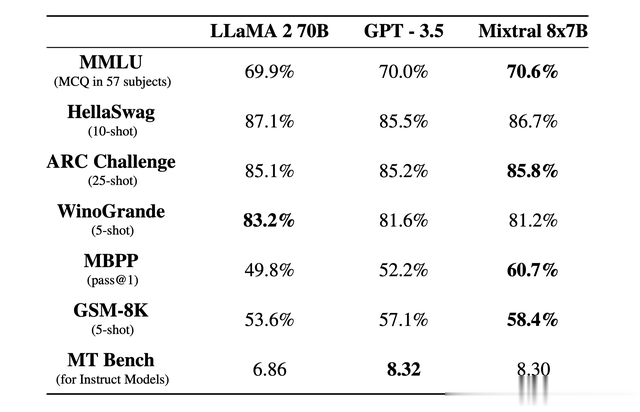
在一些下游任务上,其Mixtral模型可以跟llama2 70B模型与GPT3.5模型性能相比拟。Mixtral社区衡量了质量与推理预算。 与 Llama 2 型号相比,Mistral 7B 和 Mixtral 8x7B 属于高效模型系列。
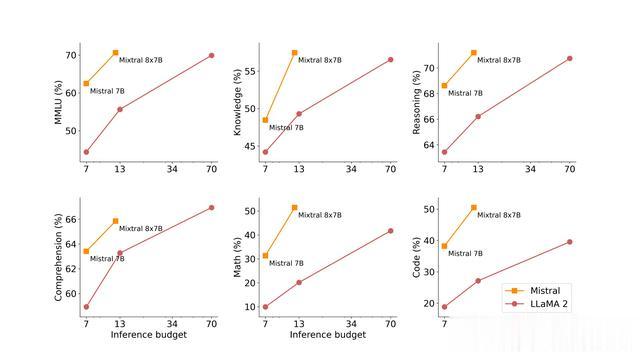
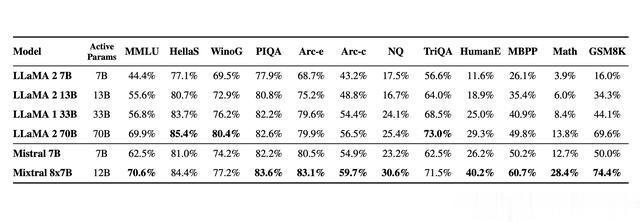
Mixtral模型释放了三个版本的模型:
Mixtral-tiny:我最具成本效益的模型,目前版本是Mistral 7B Instruct v0.2,它是 Mistral 7B Instruct 的第二个版本。 Mistral-tiny 仅适用于英语。 它在 MT-Bench 上获得 7.6 分。
Mixtral- small:该模型目前是最新模型 Mixtral 8x7B,它掌握了英语/法语/意大利语/德语/西班牙语和相关代码生成能力,并在 MT-Bench 上获得 8.3 分。
Mixtral- medium:该模型目前属于基于标准基准的顶级服务模型之一。 它掌握英语/法语/意大利语/德语/西班牙语和代码生成能力,并在 MT-Bench 上获得 8.6 分。
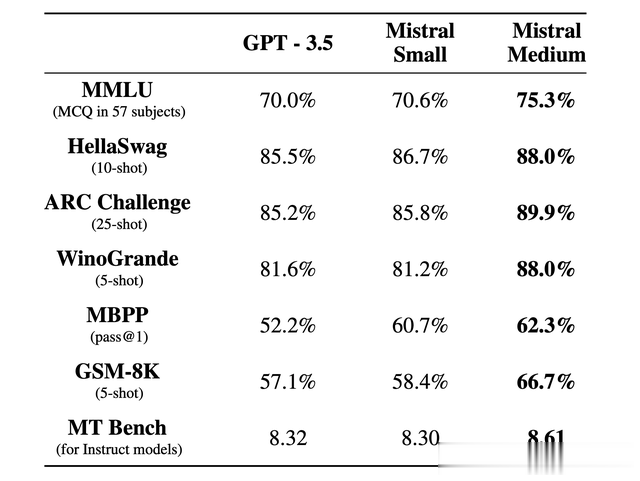
使用Mixtral模型我们可以直接使用python代码实现,这里直接使用hugging face代码实现
from transformers import AutoModelForCausalLM, AutoTokenizerdevice = "cuda" # the device to load the model ontomodel = AutoModelForCausalLM.from_pretrained("mistralai/Mixtral-8x7B-v0.1")tokenizer = AutoTokenizer.from_pretrained("mistralai/Mistral-8x7B")prompt = "My favourite condiment is"model_inputs = tokenizer([prompt], return_tensors="pt").to(device)model.to(device)generated_ids = model.generate(**model_inputs, max_new_tokens=100, do_sample=True)tokenizer.batch_decode(generated_ids)[0]当然Mixtral的预训练模型比较大,若是在自己电脑上来跑,确实需要强大的硬件支持。Mixtral社区提供了API接口调用的方式来允许用户直接使用Mixtral模型。首先你需要在Mixtral官网上订阅其服务,并获取其API。
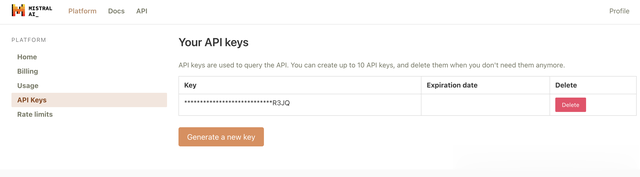
得到API key后,我们可以输入如下代码,看看都支持那些模型
pip install mistralai当然在运行代码前,需要安装mistralai库
import osfrom mistralai.client import MistralClientdef main(): api_key = "MISTRAL_API_KEY" client = MistralClient(api_key=api_key) list_models_response = client.list_models() print(list_models_response)if __name__ == "__main__": main()代码输出如下:
object='list' data=[ ModelCard(id='mistral-medium', object='model', created=1705572794, owned_by='mistralai', root=None, parent=None, permission=[ModelPermission(id='modelperm-35c4627c416e4515b878344086123148', object='model_permission', created=1705572794, allow_create_engine=False, allow_sampling=True, allow_logprobs=False, allow_search_indices=False, allow_view=True, allow_fine_tuning=False, organization='*', group=None, is_blocking=False)]), ModelCard(id='mistral-small', object='model', created=1705572794, owned_by='mistralai', root=None, parent=None, permission=[ModelPermission(id='modelperm-8b14a859d6584da7b7ee7b21c33a35b0', object='model_permission', created=1705572794, allow_create_engine=False, allow_sampling=True, allow_logprobs=False, allow_search_indices=False, allow_view=True, allow_fine_tuning=False, organization='*', group=None, is_blocking=False)]), ModelCard(id='mistral-tiny', object='model', created=1705572794, owned_by='mistralai', root=None, parent=None, permission=[ModelPermission(id='modelperm-ceae511372d8406c8a3a21c19377d9d9', object='model_permission', created=1705572794, allow_create_engine=False, allow_sampling=True, allow_logprobs=False, allow_search_indices=False, allow_view=True, allow_fine_tuning=False, organization='*', group=None, is_blocking=False)]), ModelCard(id='mistral-embed', object='model', created=1705572794, owned_by='mistralai', root=None, parent=None, permission=[ModelPermission(id='modelperm-dbd8a28c5d9d484e8b2bc484d7491104', object='model_permission', created=1705572794, allow_create_engine=False, allow_sampling=True, allow_logprobs=False, allow_search_indices=False, allow_view=True, allow_fine_tuning=False, organization='*', group=None, is_blocking=False)])]模型支持四种模型:
mistral-mediummistral-smallmistral-tinymistral-embedimport osfrom mistralai.client import MistralClientfrom mistralai.models.chat_completion import ChatMessagedef main(): api_key = "MISTRAL_API_KEY" model = "mistral-tiny" client = MistralClient(api_key=api_key) chat_response = client.chat( model=model, messages=[ChatMessage(role="user", content="What is the best French cheese?")], ) print(chat_response.choices[0].message.content)if __name__ == "__main__": main()然后,我们就可以使用API接口来使用mistral模型了,这里的api_key需要使用自己申请的key,model模型部分,可以根据自己的需求进行选择。然后输入自己的content,就可以跟模型进行交流了。当然API接口支持流式调用,这里可以修改以上代码
from mistralai.client import MistralClientfrom mistralai.models.chat_completion import ChatMessagedef main(): api_key = "MISTRAL_API_KEY" model = "mistral-tiny" client = MistralClient(api_key=api_key) for chunk in client.chat_stream( model=model, messages=[ChatMessage(role="user", content="What is the best French cheese?")], ): if chunk.choices[0].delta.content is not None: print(chunk.choices[0].delta.content, end="")if __name__ == "__main__": main()当然也可以使用asyncio进行异步调用
import asynciofrom mistralai.async_client import MistralAsyncClientfrom mistralai.models.chat_completion import ChatMessageasync def main(): api_key = "MISTRAL_API_KEY" model = "mistral-tiny" client = MistralAsyncClient(api_key=api_key) chat_response = await client.chat( model=model, messages=[ChatMessage(role="user", content="What is the best French cheese?")], ) print(chat_response.choices[0].message.content) await client.close()if __name__ == "__main__": asyncio.run(main())同样的,异步调用也可以使用流式返回
import asynciofrom mistralai.async_client import MistralAsyncClientfrom mistralai.models.chat_completion import ChatMessageasync def main(): api_key = "MISTRAL_API_KEY" model = "mistral-tiny" client = MistralAsyncClient(api_key=api_key) print("Chat response:") response = client.chat_stream( model=model, messages=[ChatMessage(role="user", content="What is the best French cheese?")], ) async for chunk in response: if chunk.choices[0].delta.content is not None: print(chunk.choices[0].delta.content, end="") print("\n") await client.close()if __name__ == "__main__": asyncio.run(main())虽然mistral模型的预训练权重可以自由下载使用,但是API接口调用是需要花钱的,毕竟是别人提供服务。若自己的电脑配置够强大,或者有自己的服务器,可以下载预训练模型,并使用transformer库,或者开源代码进行使用。
https://mistral.ai/news/mixtral-of-experts/https://github.com/mistralai/client-pythonhttps://huggingface.co/docs/transformers/model_doc/mixtralhttps://console.mistral.ai/user/api-keys/https://docs.mistral.ai/#api-access