在 ES 使用的几个内存缓存中,fielddata cache 算是一个让人头疼的家伙。
作为和 query cache 和 request cache 一样不受 GC 控制的内存使用者,fielddata cache 虽然也有 indices.fielddata.cache.size 的设置来阻止过度使用,但是默认是不限制的。
并且,当 fielddata cache 达到 indices.fielddata.cache.size 设定值的时候,虽然有类似LRU的清理算法,但是官方还是建议你进行手工清理。

那么 fielddata cache 是被什么内容使用了呢?它的作用是什么呢?我们结合官方的一些资料扒一扒。
2、fielddata 内存构成根据官方文档中的信息,我这边做了这么一个汇总。
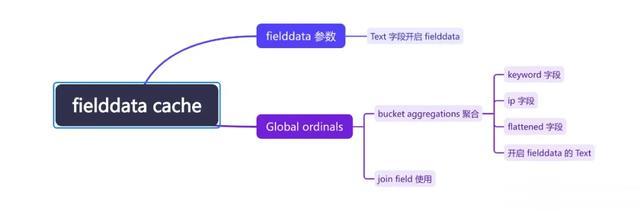
其中 bucket aggregations 特定包括我们常用的 terms 以及 composite/diversified_sampler/significant_terms。
使用的涉及面还不少,不仅有倒排字段,还有聚合查询甚至还扯上了不常见的 join 关联字段类型。感觉这类缓存的使用很复杂。
下面,我们先来看看 Text 的 fielddata。
3、Text 的 fielddata 使用Text 类型结合分词器的使用产生的倒排索引,可以满足用户进行数据检索的需求。
但是倒排索引是不支持排序聚合以及脚本编辑处理的这些需求的,而这些需求在日常业务场景下又是不避免的,那怎么办呢?
ES 做了 fielddata 这个属性来满足这一部分需求。
简单来说,开启 text 字段的 fielddata 后,ES 将把所有字段的值(即分词后的 token)装载进内存,然后对数据进行重新计算实现适合排序聚合等需求的内存数据结构。
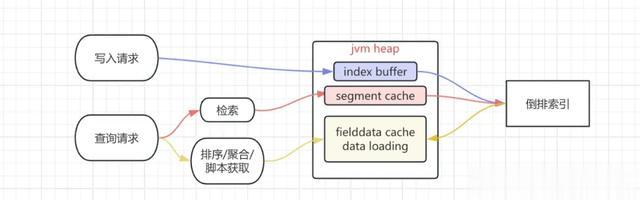
比起在检索查询直接访问写入时计算好的倒排索引数据结构,排序聚合等查询则需要在数据重载到内存中计算出数据结构。
这样的使用方式让 jvm 内的 fielddata cache 承担了较大的空间使用,动不动好几 GB。
并且在 ES 古早的版本中,由于没有 docvalue 的设计,排序聚合等场景可能需要在 text 上开启 fielddata 得以实现,不仅使用麻烦,OOM 的风险也增加了。
4、global ordinals除了 text 字段上的 fielddata,另一个使用 fielddata cache 的 global ordinals 也占据了 fielddata cache 的很多使用场景。
要想了解 global ordinals的产生,我们先聊聊 doc values。
4.1 doc values 是什么Doc values 是 lucene 4 版本开始的一个特性,它以列存的方式将字段值存储在磁盘,这样既能利用了堆外的系统缓存,同时又实现排序查询或者 scripting 所需要的数据结构,完成了 fielddata 一样的任务。
原来的写入流程和排序 scripting 是这样的
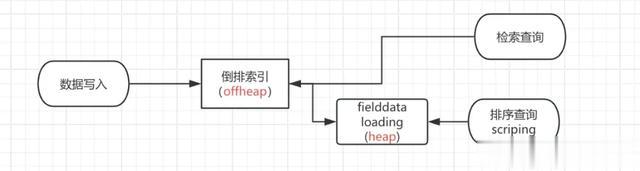
有了 doc values 后
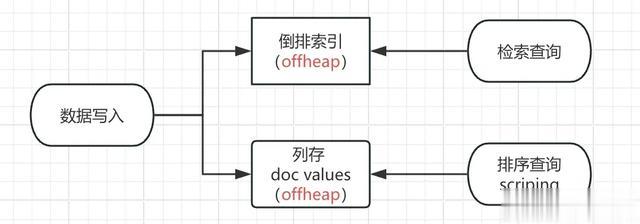
不仅可以在数据写入时就产生列存结构数据结果,同时减少了 jvm 内存的使用。
当然,如果只是为了把数据计算提前到写入时和减少对堆内内存的使用,这对于 ES 来说显然是不够的。
doc values 实际有多种存储结构,都实现了快速随机访问与数据压缩的均衡。
NumericDocValuesWriter (数值类型,ES 使用这个类型存储_seq_no等元数据字段)SortedNumericDocValuesWriter (多值内部排序的数值类型,被存储的字段是数值类型的数组,ES 使用这种方式存储用户定义的数值类型)SortedDocValuesWriter (排序的字符类型,保存原始值及 hash 位置)SortedSetDocValuesWriter (排序的字符数组类型,保存原始值及 hash 位置)对其底层实现有兴趣的同学可以查看这篇博客:https://mp.weixin.qq.com/s/kP5Pza2xRtBlcJs5WYvgjA
4.2 global ordinals 让 doc values 聚合现在我们再去理解 global ordinals 会更简单一些,下面是官网对其的定义:
To support aggregations and other operations that require looking up field values on a per-document basis, Elasticsearch uses a data structure called doc values. Term-based field types such as keyword store their doc values using an ordinal mapping for a more compact representation. This mapping works by assigning each term an incremental integer or ordinal based on its lexicographic order. The field’s doc values store only the ordinals for each document instead of the original terms, with a separate lookup structure to convert between ordinals and terms.When used during aggregations, ordinals can greatly improve performance. As an example, the terms aggregation relies only on ordinals to collect documents into buckets at the shard-level, then converts the ordinals back to their original term values when combining results across shards.Each index segment defines its own ordinal mapping, but aggregations collect data across an entire shard. So to be able to use ordinals for shard-level operations like aggregations, Elasticsearch creates a unified mapping called global ordinals. The global ordinal mapping is built on top of segment ordinals, and works by maintaining a map from global ordinal to the local ordinal for each segment.Global ordinals are used if a search contains any of the following components:Certain bucket aggregations on keyword, ip, and flattened fields. This includes terms aggregations as mentioned above, as well as composite, diversified_sampler, and significant_terms.Bucket aggregations on text fields that require fielddata to be enabled.Operations on parent and child documents from a join field, including has_child queries and parent aggregations.The global ordinal mapping uses heap memory as part of the field data cache. Aggregations on high cardinality fields can use a lot of memory and trigger the field data circuit breaker.翻译一下:
聚合涉及每个分片每个segment数据的汇总计算。以词项数据为主的字段类型(或者说字符串数据,比如 keyword 类型)的 doc values 使用一种紧凑的数据结构 ordinals 来映射原始 terms 的值,ordinals 能加速聚合查询。global ordinal 是对每个 segment oridnals 的 map 映射。global ordinal mapping 使用了 fielddata cache 的内存。注意:这里只说明是 Term-based field types,涉及的是 keyword/ip/flattened 这三个类型。其他类型比如 long/int 等使用聚合则并不会使用 global ordinals。
有兴趣的大佬可以验证 lucene 的org.apache.lucene.index.OrdinalMap 类,欢迎指正。
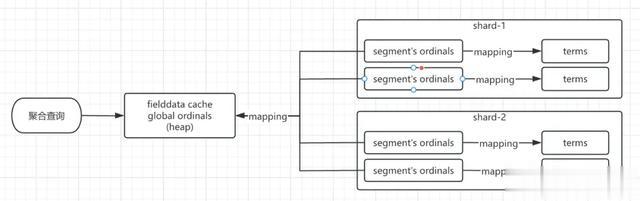
鉴于每个 segment 上的 ordinal mapping 已经压缩映射了原本的字段数据,而 global ordinals 是每个 ordinals 的一个 map。不难看出,global ordinal 在 fielddata cache 中的占用会比简单的 text 开启 fielddata 装载数据到内存减少很多。
虽然 global ordinals 已经做了不少内存使用优化,但是高基数的数据聚合会占用大量的内存,这里的高基数是指一个字段包含很大比例的唯一值。
5、 fielddata内存监控与清理5.1 设置限制这里主要有两个限制可以控制 fielddata cache 的使用:indices.fielddata.cache.size 和 indices.breaker.fielddata.limit.
indices.fielddata.cache.size(Static) The max size of the field data cache, eg 38% of node heap space, or an absolute value, eg 12GB. Defaults to unbounded. If you choose to set it, it should be smaller than Field data circuit breaker limit.indices.breaker.fielddata.limit(Dynamic) Limit for fielddata breaker. Defaults to 40% of JVM heap.indices.fielddata.cache.size 的设置要比 indices.breaker.fielddata.limit 小。
一旦超出了 breaker 的使用量,那就会触发内存的熔断了。
这里做个假设吧,一个 ES 进程实例申请 jvm 内存 10GB,那么 indices.breaker.fielddata.limit 就默认为 heap 的 40%,也就是 4GB,这样 indices.fielddata.cache.size 的设定则要小于 40% 或者 4GB,比如 35% 或者 3.5GB,那么就会有 0.5GB 的 indices.breaker.fielddata.limit 冗余。
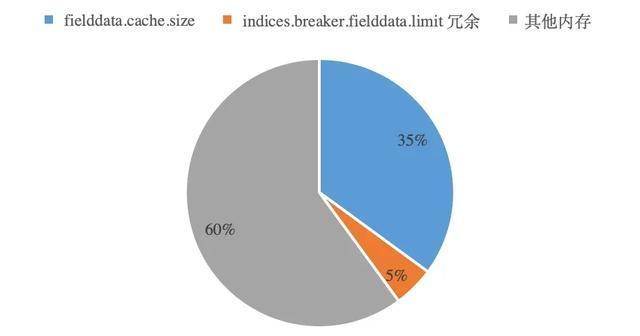
监控命令
GET _cat/fielddata?v#查看索引的fielddataGET _stats/fielddata?level=indices#查看主机级别GET _nodes/stats/indices/fielddata?fields=*#查看主机上index级别字段级别GET _nodes/stats/indices/fielddata?level=indices&fields=*为了避免监控数据膨胀,一般是做到索引级别的使用监控,实际定位问题去使用字段级别的维度去人工定位。
清理命令
POST /my-index-000001/_cache/clear?fielddata=true6、 小结和建议不难看出,doc values 其实是对倒排索引的 fielddata 使用的变迁和优化,以一种列存的文件模式实现了聚合排序场景的需求使用。
Text 开启 fielddata 的实现方式相对比较粗糙,简单的申请 jvm 内存进行多个场景的数据构建计算,不仅需要不少的内存资源,也无法利用更多的计算资源和空间。
Doc values 则经过各种列存数据文件的精细设计,即满足了 fielddata 的需求场景,也降低了 jvm 内存的使用,又在数据压缩和快速读取上实现了最大化,替代 fielddata 大部分的使用场景。
但对于 text 文本字符串长文中的单字聚合场景,比如:实现 text 字段的词云效果,还必须使用 fielddata enable 属性。
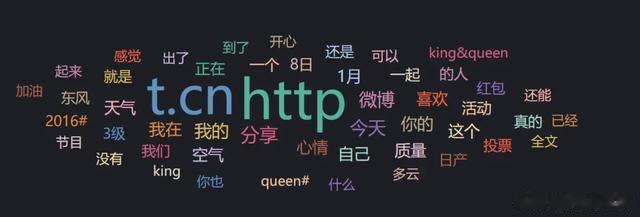
Kibana 8.X 如何做出靠谱的词云图?
其实不管是 fielddata 还是 doc values,ES 使用 fielddata cache 几乎都是满足聚合排序等查询需求(除 join 字段)。
但是 jvm heap 内存资源是紧张且宝贵的,为了安全使用 ES,加强堆内的资源进行有效的利用,在此总结了以下几个建议:
合理设置 indices.fielddata.cache.size避免对 text 直接使用 fielddata,利用 doc values 替代其使用场景。建立模型,清洗数据,利用合适的字段进行排序聚合。比如 数字类型数据双字段,聚合使用 long,查询匹配使用 keyword。terms/sampler/significant_terms 这些聚合方法中将 execution_hint 设置为 map,一般基数在百万以下的改为 map 方式会更优(字段值特别长的例外)。可以考虑对没有更新的历史数据进行 forcemerge,将 segment 数量减少到最小。尽量减少 ES 执行聚合的复杂度,超大数据体量多层次的聚合还是对系统资源的一次考验。做好对 fielddata 内存的监控和及时的处理,有必要时直接清理7、 参考资料DocValues 存储格式及压缩实现
https://mp.weixin.qq.com/s/kP5Pza2xRtBlcJs5WYvgjA
Disk-Based Field Data a.k.a. Doc Values
https://www.elastic.co/cn/blog/disk-based-field-data-a-k-a-doc-values
Doc Values and Fielddata
https://www.elastic.co/guide/en/elasticsearch/guide/current/docvalues-and-fielddata.html
作者:金多安
来源-微信公众号:铭毅天下Elasticsearch
出处:https://mp.weixin.qq.com/s/o_gB7smf12IvNYx8HVW3Gg