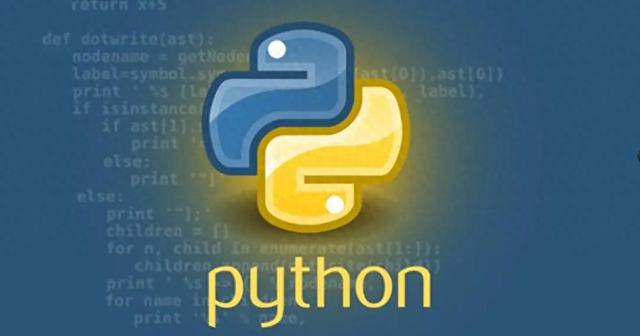
大家好,今天为大家分享一个超强的 Python 库 - optuna。
Github地址:https://github.com/optuna/optuna
超参数优化是机器学习中的重要问题,它涉及在训练模型时选择最优的超参数组合,以提高模型的性能和泛化能力。Optuna是一个用于自动化超参数优化的库,它提供了有效的参数搜索算法和方便的结果可视化工具。
安装与基本使用可以通过pip安装Optuna库:
pip install optuna以下是一个简单的示例,展示如何使用Optuna进行超参数优化:
import optunadef objective(trial): x = trial.suggest_float('x', -10, 10) return (x - 2) ** 2 # 目标是最小化 (x - 2) ** 2study = optuna.create_study()study.optimize(objective, n_trials=100)print(study.best_params)定义优化目标函数定义优化目标函数是Optuna中的关键步骤。这个函数的返回值将作为优化的目标。
def objective(trial): # 定义超参数 learning_rate = trial.suggest_float('learning_rate', 1e-5, 1e-2, log=True) num_layers = trial.suggest_int('num_layers', 1, 3) # 使用超参数训练模型 model = train_model(learning_rate, num_layers) # 计算模型性能指标 score = evaluate_model(model) return score定义搜索空间Optuna可以定义超参数的搜索空间,包括连续型、离散型和条件型参数。
def objective(trial): # 定义搜索空间 learning_rate = trial.suggest_float('learning_rate', 1e-5, 1e-2, log=True) num_layers = trial.suggest_int('num_layers', 1, 3) activation = trial.suggest_categorical('activation', ['relu', 'tanh', 'sigmoid']) return score选择搜索算法Optuna提供了多种搜索算法,默认使用TPE算法。也可以选择其他搜索算法。
study = optuna.create_study(direction='maximize', sampler=optuna.samplers.RandomSampler())并行化优化Optuna支持并行化优化,可以加速优化过程。可以使用多线程、多进程或分布式优化。
study.optimize(objective, n_trials=100, n_jobs=4)结果分析与可视化Optuna提供了丰富的结果分析和可视化工具,帮助理解优化结果并调整搜索策略。
optuna.visualization.plot_optimization_history(study)optuna.visualization.plot_parallel_coordinate(study)高级主题与应用场景1、多目标优化在实际问题中,往往存在多个目标需要优化,例如在模型训练中既希望减小训练误差,又希望减小模型复杂度。
Optuna提供了多目标优化的支持,可以同时优化多个目标函数。
示例代码:
def objective(trial): x = trial.suggest_float('x', -10, 10) y = trial.suggest_float('y', -10, 10) # 优化目标函数为 x^2 + y^2 和 (x-2)^2 + (y-2)^2 return x**2 + y**2, (x-2)**2 + (y-2)**2study = optuna.create_study(directions=['minimize', 'minimize'])study.optimize(objective, n_trials=100)2、 集成OptunaOptuna可以与其他机器学习框架(如PyTorch、TensorFlow等)无缝集成,以实现超参数优化。
以下是一个使用Optuna优化PyTorch模型的示例:
import torchimport torch.nn as nnimport torch.optim as optimimport optunadef objective(trial): # 定义超参数 learning_rate = trial.suggest_float('learning_rate', 1e-5, 1e-2, log=True) num_hidden_units = trial.suggest_int('num_hidden_units', 16, 256, log=True) # 创建模型 model = nn.Sequential( nn.Linear(784, num_hidden_units), nn.ReLU(), nn.Linear(num_hidden_units, 10) ) # 定义损失函数和优化器 criterion = nn.CrossEntropyLoss() optimizer = optim.SGD(model.parameters(), lr=learning_rate) # 训练模型 for epoch in range(num_epochs): for inputs, labels in train_loader: optimizer.zero_grad() outputs = model(inputs) loss = criterion(outputs, labels) loss.backward() optimizer.step() # 返回验证集上的准确率作为优化目标 accuracy = evaluate_model(model, val_loader) return accuracystudy = optuna.create_study()study.optimize(objective, n_trials=100)示例代码以下是一个完整的示例代码,演示了如何使用Optuna进行超参数优化的完整过程。在这个示例中,将使用Optuna来优化一个简单的支持向量机(SVM)模型的超参数。
import optunafrom sklearn.datasets import load_irisfrom sklearn.model_selection import train_test_splitfrom sklearn.svm import SVCfrom sklearn.metrics import accuracy_score# 加载数据集iris = load_iris()X, y = iris.data, iris.target# 划分训练集和验证集X_train, X_val, y_train, y_val = train_test_split(X, y, test_size=0.2, random_state=42)# 定义优化目标函数def objective(trial): # 定义超参数搜索空间 C = trial.suggest_loguniform('C', 1e-4, 1e4) gamma = trial.suggest_loguniform('gamma', 1e-4, 1e4) kernel = trial.suggest_categorical('kernel', ['linear', 'rbf', 'poly']) # 创建SVM模型 model = SVC(C=C, gamma=gamma, kernel=kernel) # 在训练集上训练模型 model.fit(X_train, y_train) # 在验证集上评估模型 y_pred = model.predict(X_val) accuracy = accuracy_score(y_val, y_pred) return accuracy# 创建Optuna study对象study = optuna.create_study(direction='maximize')# 运行优化过程study.optimize(objective, n_trials=100)# 输出最佳超参数组合print("Best hyperparameters: ", study.best_params)# 输出最佳验证集准确率print("Best validation accuracy: ", study.best_value)在这个示例中,首先加载了一个经典的鸢尾花数据集,然后将数据集划分为训练集和验证集。接着,定义了一个优化目标函数,该函数接受一个Trial对象作为参数,从中获取超参数的建议值,并使用这些超参数训练一个SVM模型,并在验证集上评估模型的准确率。最后,使用Optuna的create_study()函数创建一个study对象,并调用optimize()函数来运行优化过程,指定要运行的试验次数。优化完成后,输出了找到的最佳超参数组合以及对应的验证集准确率。
总结Optuna是一个强大的超参数优化框架,为机器学习任务提供了自动化的参数搜索和优化功能。通过定义优化目标函数和搜索空间,Optuna可以帮助用户快速找到最优的超参数组合,从而提高模型的性能和泛化能力。其丰富的搜索算法和可视化工具使得超参数优化过程更加高效和可控。Optuna的简单易用性和灵活性使得它成为了机器学习工程师和研究人员的首选工具之一。